Predicting relapse in breast cancer using multimodal data
Primary supervisor: Claude Chelala, Queen Mary University of London
Secondary supervisor: Rifat Hamoudi, UCL
Project
Although breast cancer is detected earlier than ever and breast-conserving surgery is an effective cure for many, relapse rates are high despite apparently tumour-free surgical margins. Whether this is due to pre-existing residual microscopic disease or the development of new molecular changes in the remaining tissue is unclear. Our bulk RNAseq analysis of matched tumour and histologically normal (HN) tissues
from young breast cancer patients suggested that even HN tissues up to 10 cm from the primary lesion frequently harbour molecular aberrations [1] (Fig 1a). We identified four distinct gene expression signatures in HN tissues, with the metabolic type strongly associated with poor prognosis (HR 6.1, p<0.001) (Fig 1b,c). Since disease severity is primarily evaluated using histological analysis, these findings present an opportunity to:
- Better define excision margins in breast-conserving surgery,
- Understand otherwise undetectable early neoplastic change,
- Predict risk of early recurrence.
Aims
- Refine our signature using spatial transcriptomics at high resolution in fresh frozen tissues from patients with tumour, proximal ‘normal’, distal ‘normal’ (Fig 1) and contralateral ‘normal’ samples. This will determine the precise areas of molecular aberration and whether this is latent throughout either or both breasts. (Data: RNAseq).
- Incorporate deep learning-based methods to extract clinically-relevant features using diagnostic and/or whole slide H&Es, and radiological imaging data to develop and train a computational model to detect the high-risk molecular subtype in the histopathological patterns of digital images and facilitate the identification of patients at increased risk of recurrence. (Data: digital pathology).
- Validate and test the derived digital pathology model using artificial intelligence to interrogate the clinical images for >10k patients in combination with follow-up data from electronic health records (comorbidities, symptoms, tests, diagnoses). This will aid stratification of patients based on risk of recurrence and determine the potential for this approach to improve clinical screening tests at the population level, without expensive molecular data [2]. (Data: clinical).
- Link derived risk predictions with tumour and germline whole genome sequencing (WGS) data on available patients (n>200). Using genetic ancestry measures, we have recently shown that non-European patients present earlier and die younger, and that there are marked differences in germline and somatic mutation rates and profiles of European, Black and South Asian women with breast cancer [3]. These include several therapeutic targets with varying potential effectiveness in different genetic backgrounds. (Data: genomic).
By combining spatial transcriptomics with artificial intelligence-trained digital pathology, genomics and extensive clinical data in a multi-modal approach, we aim to improve early identification of high-risk patients, determined by ethnicity and/or molecular subtype.
Key resources
- Training: The national Breast Cancer Now Biobank (BCN Biobank), hosted at BCI, provides access to >120k samples from >10k donors, with extensive clinical follow-up, and digitised H&E and radiological imaging.
- Validation: Curated TCGA breast cancer cohort: n=1,076 patients from various subtypes of breast cancer together with associated H&E and radiological images.
- Matched clinical follow-up, imaging, and tumour and germline WGS data for ≥200 patients.
- NVIDIA GPU A100 high performance computing cluster supports running deep learning models.
Candidate background
This project combines computational analysis and synthesis of spatial transcriptomics, genomics and high-dimensional imaging data with extensive quantitative/qualitative clinical data from electronic health records. We therefore invite applications from candidates with a background in quantitative cancer biology (e.g. statistics, computation, data analytics). Demonstrated proficiency in Python and familiarity with the Unix/Linux environment is essential; familiarity with R and high-performance computing clusters is desirable.
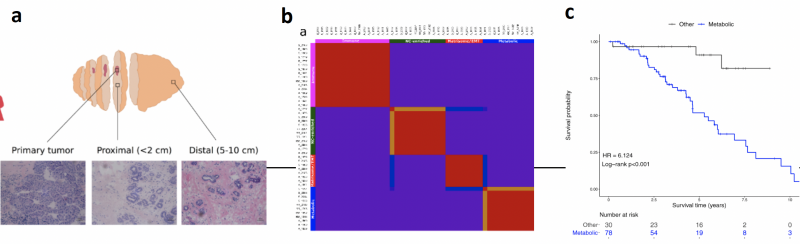
Potential Research Placements
- Oscar Carlos Maiques, Barts Cancer Institute, Queen Mary University of London
- Vivek Singh, Barts Cancer Institute, Queen Mary University of London
- Rifat Hamoudi, Surgical Biotechnology, University College London
References
- Gadaleta et al., npj Breast Cancer, 2020, https://doi.org/10.1038/s41523-020-00182-9.
- Arslan et al., Commun Med (Lond), 2024, https://www.nature.com/articles/s43856-024-00471-5
- Thorn, Gadaleta et al. Preprint: medRxiv 2024.05.15.24307435; under review Nat Comms.